Data-Driven Insights for Smarter Insurance Risk Management
.png)
The insurance industry is rapidly embracing advanced analytics to propel business growth and enhance operational efficiency. As companies navigate the complexities of risk assessment and customer service, the reliance on data-driven strategies are becoming paramount.
In 2023, the market for insurance analytics reached USD 12.65 billion, highlighting the sector's commitment to adopting these technologies. This trend is set to continue, with a projected market value of USD 14.50 billion in 2024 and an impressive USD 44.77 billion by 2032. This surge is transforming how insurers operate, making processes more streamlined and decision-making more precise.
In this blog post, we’ll delve deeper into insurance data analytics and its role in improving insurance services.
What is insurance analytics?
Insurance analytics refers to the process of collecting, analyzing, and interpreting insurance-related data to generate actionable insights that help drive business decisions and improve overall performance.
It involves analyzing vast amounts of data from various sources such as customer interactions, policy details, claims history, and external sources like social media and credit reports. By leveraging analytics, insurance companies can better understand risks, set accurate pricing, personalize their services, and detect fraud more effectively.
The importance of insurance data analytics for your business
Insurance analytics goes beyond optimizing day-to-day operations; it strategically positions your business for sustainable growth and resilience in a competitive marketplace. Here’s how each aspect of insurance analytics can significantly benefit your business:
- Better risk evaluation: Analyze historical data and identify patterns to enable more accurate risk assessment. This helps with effective pricing and managing reserves for potential payouts.
- Improved lead generation: Analyze demographic data and behavioral insights to pinpoint potential customers who match your ideal policyholder profile. This targeted approach refines marketing campaigns and increases conversion rates.
- Fraud detection: Advanced analytics approaches, like machine learning and pattern recognition, help detect unusual behaviors or anomalies in claims or applications that could indicate fraudulent activity. Early detection saves significant costs and helps maintain the integrity of operations.
- Personalized pricing: Analytics allows for personalized pricing models based on individual risk assessments. By considering factors unique to each customer, such as their claims history and lifestyle choices, insurers can offer tailored pricing that is fair and competitive.
- Enhanced customer experience: Using customer data, insurers can enhance customer service and create more personalized interactions. Analytics also enables proactive communication and tailored product offerings, improving the overall customer experience.
- Competitive advantage: Analytics empowers smarter decision-making, reduced costs, improved efficiency, and enhanced customer service, providing a significant edge over competitors. Companies that leverage analytics can adapt more quickly to market changes and customer needs, maintaining a leading position in the industry.
The different types of insurance analytics
Insurance analytics can be categorized into several types, each focusing on different aspects of the insurance business.
- Descriptive analytics
This form of analytics focuses on summarizing historical data to understand past trends.
For example, analyzing monthly claims data over the past five years can reveal peak claim periods or demographics with high claim rates.
- Diagnostic analytics
This type involves deeper analysis to understand why certain events occurred. It uses techniques like drill-down, data discovery, correlations, and causality analysis to find the reasons behind trends or unexpected outcomes.
For example, after noticing a spike in claims during a particular quarter, an insurer might use diagnostic analytics to analyze which specific policies or geographic areas contributed to the spike and determine if external factors like weather events or economic changes played a role.
- Predictive analytics
It uses historical data to model and forecast future events. This is crucial for proactive risk management and optimizing pricing strategies.
For example, an auto insurance company uses historical data about accidents, driver profiles, and vehicle types to predict which policyholders are more likely to file a claim.
- Prescriptive analytics
This type of analytics goes beyond predicting future outcomes by also suggesting actions to benefit from the predictions.
For example, using predictive models that identify areas with high claims numbers, an insurer could implement specific risk-reduction measures. These include increasing safety measures in those regions or changing the terms of policies to better handle potential losses.
- Customer analytics
This focuses on analyzing customer behavior, preferences, and satisfaction to tailor products, optimize marketing campaigns, improve customer retention, and enhance overall customer service.
For example, using data from customer interactions, feedback, and behavior, a life insurance company can identify common factors among customers who lapse on their policies. It can then develop targeted retention strategies, such as personalized communication or special offers, to retain high-risk customers.
- Risk analytics
Specifically focused on identifying, measuring, and managing risk, this type of analytics is integral to underwriting and claims management.
For example, an insurer can use risk analytics to assess the potential risk of insuring homes in a coastal area prone to hurricanes. By analyzing historical data on weather patterns and past claims, the company can determine premium prices that accurately reflect the level of risk.
- Cognitive analytics
Leveraging artificial intelligence (AI) and machine learning, cognitive analytics can interpret natural language and learn from data in a way that mimics human thought processes.
For example, a health insurance company employs a chatbot that uses cognitive analytics to understand and respond to customer inquiries naturally. The bot can assist in processing claims or provide personalized advice on coverage options based on the customer’s spoken or typed messages.
Uses of insurance analytics
Insurance data analytics empowers insurers in several key areas.
- Claims management and automation: Analytics can streamline the claims process by automating tasks like claims triage, damage assessment, and payout calculations. This speeds up claims settlement and improves customer satisfaction.
- Customer segmentation and targeted marketing: By analyzing customer data, insurers can better understand policyholder behavior, preferences, and lifetime value. This enables targeted marketing campaigns, personalized product recommendations, and improved customer retention.
- Underwriting automation and optimization: Machine learning models can automate and accelerate the underwriting process, particularly for simpler risks. Analytics helps underwriters focus on complex cases while improving risk selection and policy pricing.
- Product development and innovation: Analytics provides insights into emerging customer needs and market trends. This guides the development of new insurance products and features that better meet evolving demands. For example, insurers might leverage analytics to develop innovative products like usage-based car insurance or personalized health plans.
- Operational efficiency and cost reduction: By automating processes, predicting trends, and optimizing resource allocation, analytics helps insurers streamline operations and reduce costs across the value chain.
- Comprehensive overview of business: Insurance analytics offers a comprehensive overview of business operations by analyzing data across various domains, from risk assessment and claims processing to customer behavior and market trends. This deep insight enables insurers to make data-driven decisions, optimize performance, and enhance strategic planning.
Streamline insurance analytics with DataBrain
To fully utilize insurance data, organizations require embedded analytics software that integrates smoothly with existing tools and systems.
DataBrain offers embedded dashboards that simplify complex insurance data analysis. It features real-time data analytics and straightforward plug-and-play integrations designed to elevate insurance operations.
The platform not only provides snapshots of current metrics but also delivers deep insights that enable trend predictions and informed decision-making.
DataBrain addresses several challenges mentioned throughout this post:
- Simplifying complex data analysis: DataBrain breaks down complex insurance data into manageable insights. The platform facilitates real-time monitoring and analysis, making it easier for insurance managers to operate more effectively and efficiently.
- Customization and integration: The platform stands out with its highly customizable dashboards and reporting tools, which can be tailored to meet the specific needs of any department. Its seamless embedding capabilities allow analytics integration into the insurance applications already in use, facilitating a cohesive and integrated user experience.
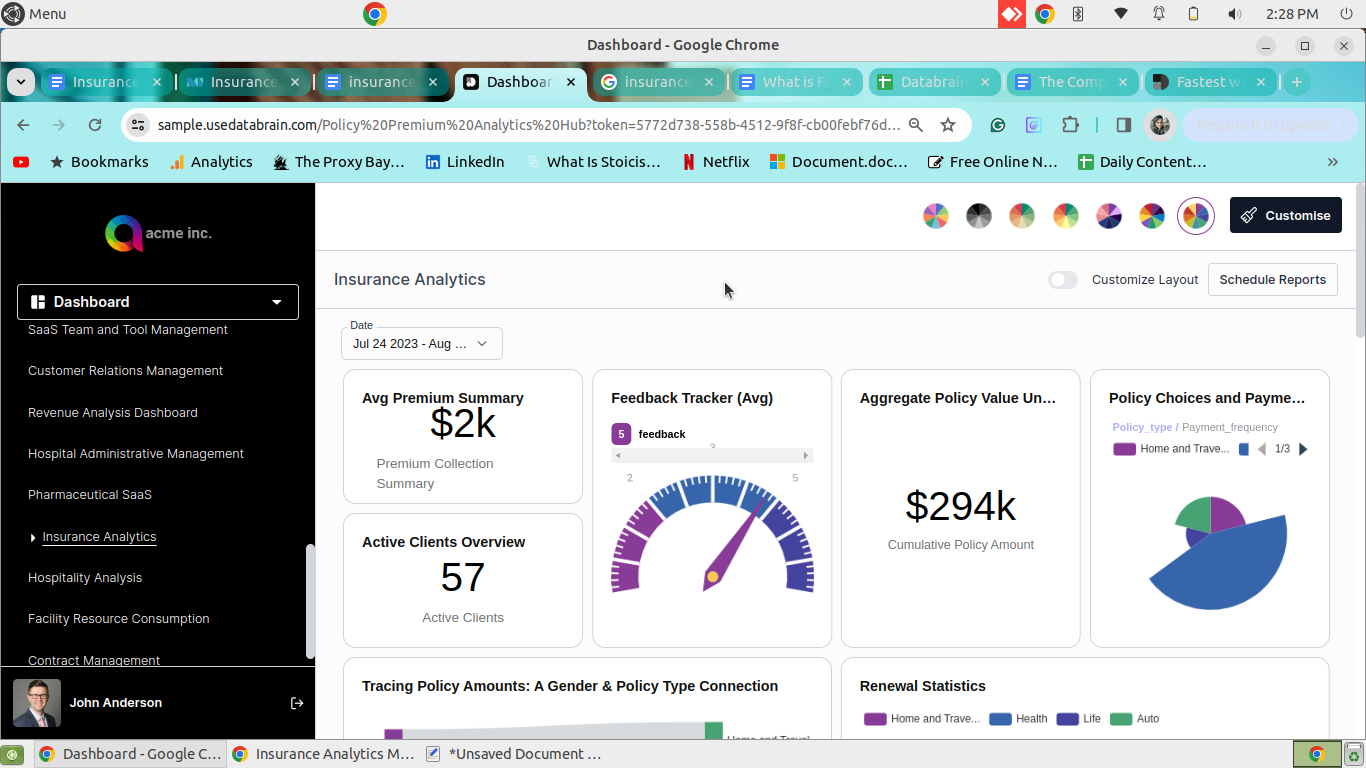
- Efficient setup and immediate impact: Engineered for quick deployment, DataBrain minimizes the delay between installation and utilization. Pre-configured templates for common tasks and a low-code environment reduce the need for technical expertise. This speeds up the customization process and delivers immediate benefits.
- Interactive analytics: Dive into DataBrain’s interactive insurance analytics dashboard to explore key analytics in a dynamic and engaging way. This tool helps unlock new perspectives on insurance data, facilitating more informed decision-making and a deeper understanding of industry trends.
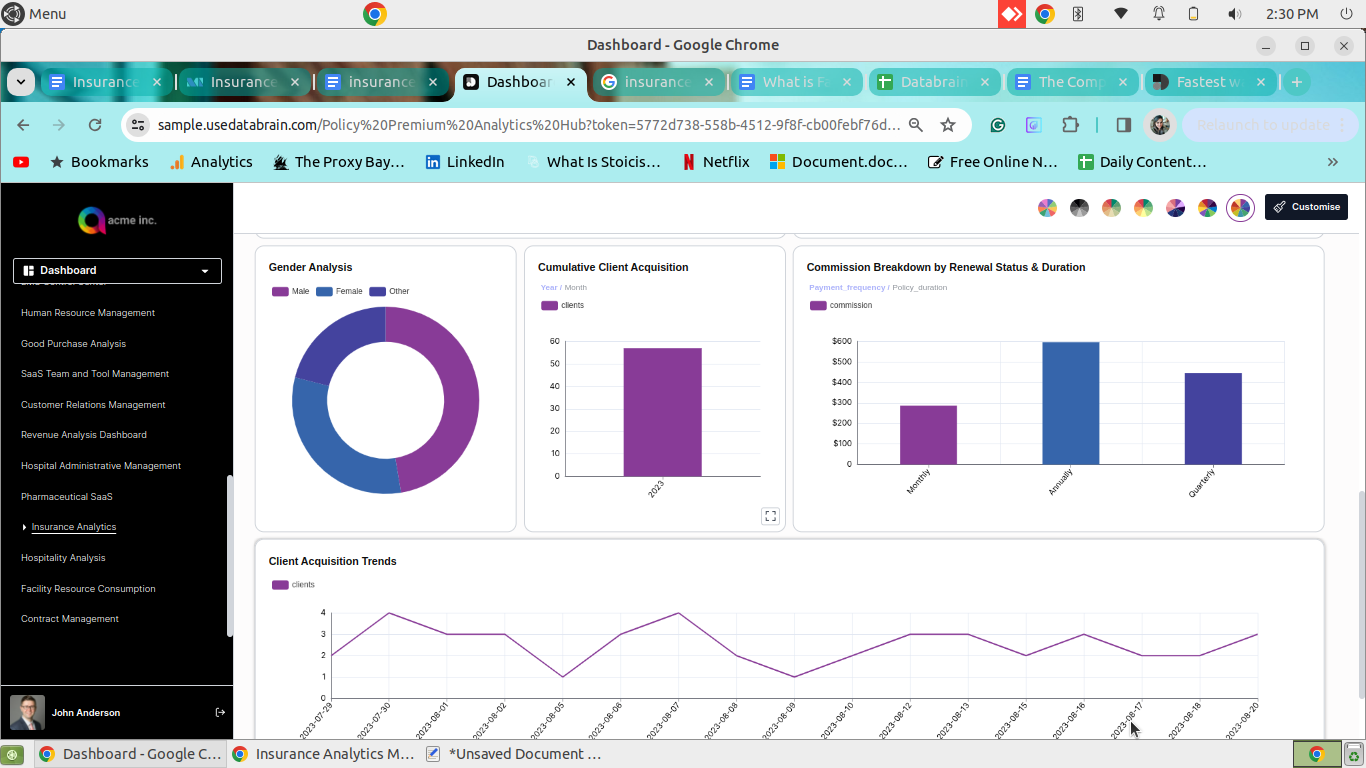
Unlock the full potential of your insurance data with DataBrain's embedded analytics. Transform your organizational strategies, make data-driven decisions, and gain comprehensive insights into your operations. Start exploring with DataBrain’s interactive dashboard today and take the first step toward a more informed and effective management approach!